Welcome to 6G@HK
Seminars & Talks
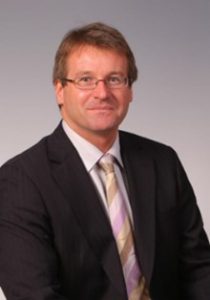
Creating New Radio Frequency Wave Technology for 6G
Abstract:Â Radio frequency (RF) waves are a fundamental phenomenon that can carry electromagnetic signals and energy through space and interact with it. Their use in wireless communication has revolutionized our lives and created a mobile information society and new industries. However, RF wave technology can do much more and in this talk I explore new RF wave technologies that can be further exploited for 6G. This includes the way we monitor and image the environment, monitor our health, navigate, transfer energy, harness existing waves and utilize the Internet of Things (IoT). These new applications can broadly be classified as wave shaping, wave sensing and wave characterization. Furthermore, new fundamental breakthroughs in wave theory such as metamaterials, space-time structures, time-reversal and wave sensing can lead to even more developments. While each of these technologies and fundamental breakthroughs are promising, significant further research is needed to exploit the enormous potential of new RF wave technology for 6G. Slide
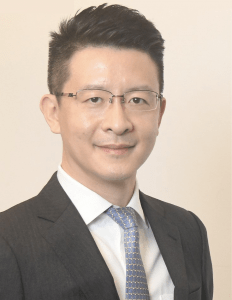
Memristor Empowered Ultra-fast Baseband Processing
Abstract: To support emerging applications ranging from holographic communications to extended reality, next-generation mobile wireless communication systems require ultra-fast and energy-efficient (UFEE) baseband processors. Traditional complementary metal-oxide-semiconductor (CMOS)-based baseband processors and computing at large face two challenges, transistor scaling and the von Neumann bottleneck. The former is caused by approaching transistors’ physical limits and the latter by energy-consuming data shuffling between memory and processors. Recently, implemented using arrays of memristors (i.e., programmable resistors), in-memory computing, which features co-located storage and computing, has emerged to a promising solution for tackling the two challenges and thereby caused excitements in the areas of ultra-fast AI and scientific computing. In this talk, I will report the first attempt on designing in-memory computing empowered baseband processing for the widely adopted multiple-input-multiple-output orthogonal frequency division multiplexing (MIMO-OFDM) air interface. The key operations, including discrete Fourier transform, linear MIMO detection, and channel estimation, are realized using arrays of resistive random-access memory (RRAM), a popular type of memristor, thereby supporting one-step execution. By prototyping and simulations, I will demonstrate that the RRAM-based communication system can significantly outperform its CMOS-based counterpart in terms of speed and energy efficiency by 10^3 and 10^6 times, respectively. The results pave a potential pathway for RRAM-based in-memory computing to enable UFEE 6G communications. Slide
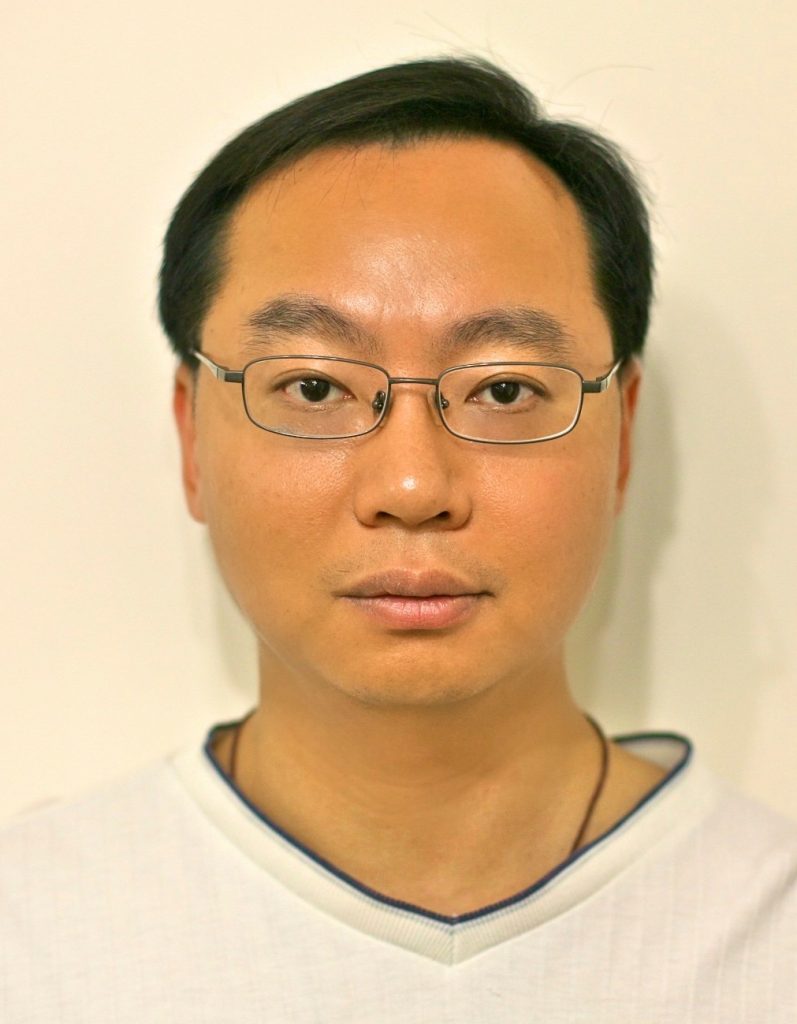
Online DNN for Massive MIMO Channel Estimation
Abstract: Massive MIMO systems can achieve high spectral efficiency as well as interference mitigation through spatial multiplexing and spatial filtering. Channel estimation (CSIR) and feedback (CSIT) of Massive MIMO systems is a critical but challenging component to realize these potential gains. For instance, conventional channel estimation solution requires pilot overhead, which scales with number of transmit antennas. In order to decrease the pilot overheads, we will need to exploit the underlying sparsity structures of massive MIMO channels. There are many works that consider exploiting different levels of sparsity (such as link level sparsity and multi-user sparsity) in the channel estimation and channel feedbacks. However, these solutions are iterative and cannot be implemented on a real-time basis. Recently, there are some works that utilize DNN to achieve real-time inferencing of these iterative CE algorithms. However, the training of these DNN solutions require labeled data (knowledge of true channel) and hence, they are trained in an offline manner based on the channel samples generated from simulations. As such, the offline training will suffer from potential model mismatch because the actual scattering environment the mobile sees will surely be different from that assumed in the simulation model. In this talk, we introduce an online training framework for real-time implementation of the DNN for channel estimation and feedbacks in massive MIMO systems. We first consider a point-to-point system and extend the framework to multi-user systems. The proposed online DNN can track the actual propagation environment on a real-time basis without prior knowledge of the true channel matrix and therefore, is robust to different propagation models, antenna geometry as well as the underlying non-linearity. Slide
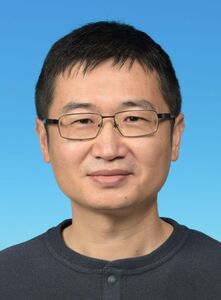
The Reasonable Effectiveness of Graph Neural Networks for Wireless Communications
Abstract: Deep learning techniques have been recently applied to solve various challenging problems in wireless communications. The effectiveness of such approaches highly depends on the neural network architecture. Early attempts adopted architectures inherited from such applications as computer vision, e.g., multi-layer perceptrons (MLPs) and convolutional neural networks (CNNs). Unfortunately, methods based on these classic architectures often require huge amounts of training samples (i.e., poor generalization), and yield poor performance in large-scale networks (i.e., poor scalability). This talk will introduce the graph neural network (GNN) as a promising neural architecture to solve generic design problems in wireless communications. It will present a theoretical analysis to quantify the performance gains of GNNs over unstructured neural networks, in terms of generalization performance and sample complexity. Moreover, effective design guidelines for the GNN architecture will also be presented, accompanied with various examples. Slide